Aircraft mission analysis enhancement by using data science and machine learning
- Junghyun (Andy) Kim
- Dec 31, 2019
- 1 min read
Updated: Dec 9, 2024
Sponsored Research (Federal Aviation Administration)
Research Motivation
As aviation traffic continues to grow, many airlines have increasing concerns about reducing (or optimizing) fuel consumption because fuel accounts for up to 40% of airline operating costs. To that end, it is pre-requisite to estimate fuel consumption as accurate as possible. However, most of the aircraft operation datasets have been elusive due to proprietary purposes. Under these circumstances, a comprehensive software named Aviation Environmental Design Tool (AEDT) has been prevalently used by many aerospace engineers to calculate the fuel consumption. It is highly hypothesized that the fidelity of AEDT would be enhanced given the AEDT could collaborate with reliable weather information.
Key Idea
I propose a novel aircraft mission analysis framework by incorporating data-driven approaches with the AEDT and machine learning techniques to improve the accuracy of aircraft mission analysis.
Overview of the proposed methodology
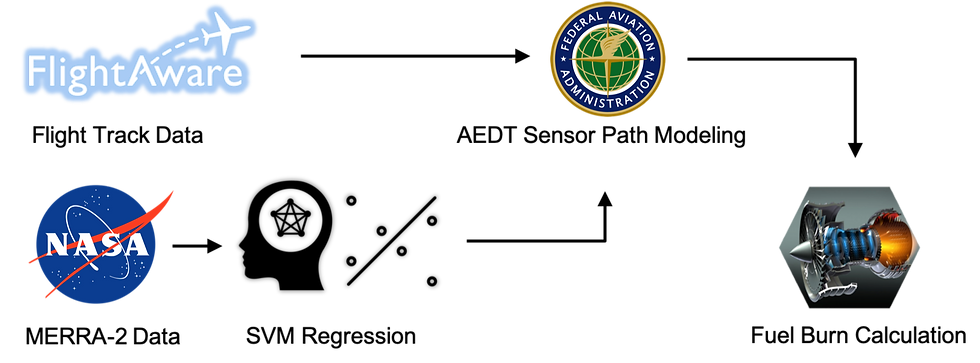
Results
The results show that the collaboration of the Support Vector Machine (SVM) weather regression model and the AEDT precisely matched the fuel consumption reference data.
Publication
Click here to access the publication.
Comments