Collaborative Research (Kyungpook National University)
Research Motivation
As aviation traffic continues to grow, most airport operators are concerned about ground delays, which are directly related to operating costs. To systematically manage the daily airport operations of the United States, the operators typically classify the operations into three categories: 1) good days, 2) average days, and 3) bad days. Among various factors in the ground delay program, wind speed at an airport is one of the most significant factors to assess efficiency. While accurate wind information is imperative for airport operators, wind forecasting has been considered as one of the most challenging tasks in the aviation industry.
Key Idea
This research propose a hybrid approach that combines a machine learning technique with the Kalman filtering for a wind nowcasting problem in the aviation industry. More specficially, this research has three objectives: 1) compare machine learning models (i.e., MLP, GP, and LSTM) to identify the most appropriate model for wind nowcasting, 2) combine the selected machine learning model with an unscented Kalman filter (UKF) to improve the model fidelity, and 3) perform the Monte Carlo simulation to quantify uncertainties that arise from the modeling process.
Overview of the proposed methodology
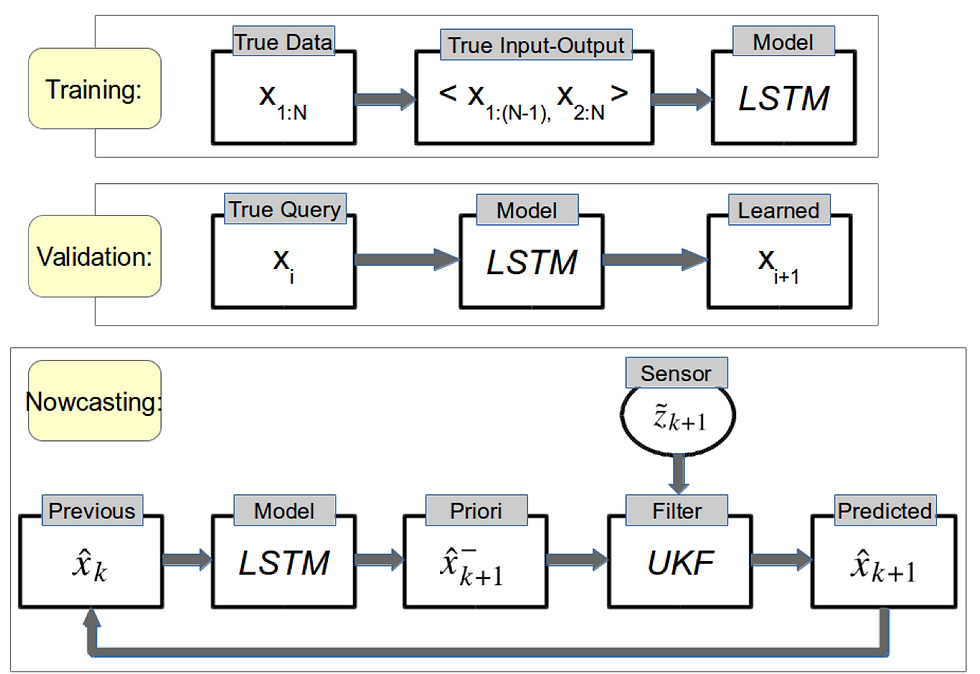
Results
The results show that time-series wind data are best predicted by the LSTM compared to the other machine learning models and the UKF-aided LSTM approach outperforms the LSTM, especially if it must account for long-term wind forecasting.
UKF-aided LSTM vs. LSTM only
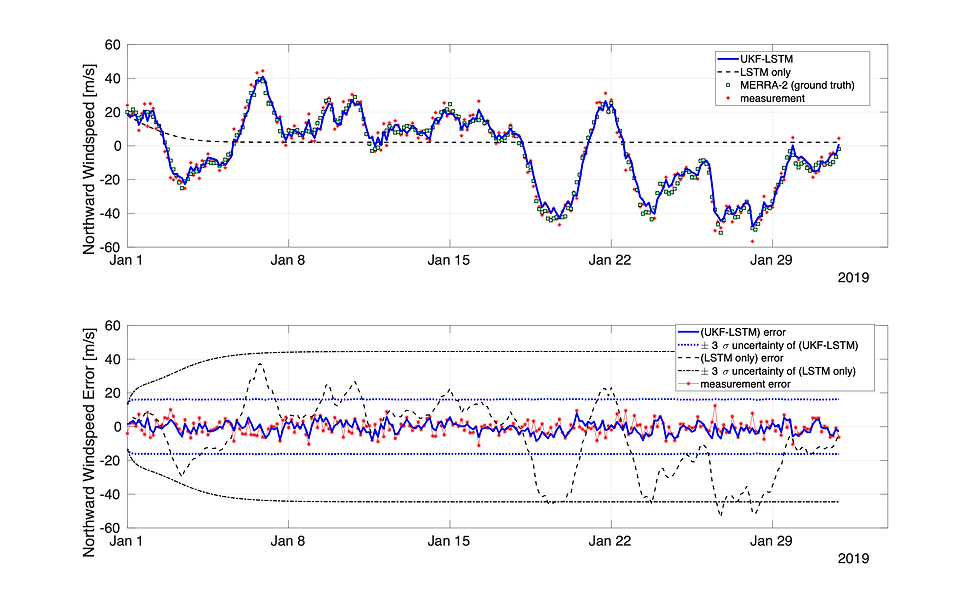
Publication
Click here to access the publication
Comentários